This editorial was first featured in Pharmafocus.
Pharmafocus provides pharmaceutical industry news, analysis and insights. Pharmafocus, January/February 2024, pages [14-15] © Samedan Ltd.
Artificial intelligence: Selective boom?
Artificial intelligence (AI) is a relatively young science — the field is actually younger than 100 years — and yet its recent acceleration belies any concern we might have over its adoption. The buzz around AI today typically centers on its impact on business as a trade-off between productivity improvement and job displacement. But what about its impact on science? Is science also experiencing the biggest AI boom to date?
Research published in 2020 in the American Economic Review (which included broad scientific fields such as agricultural crop yields in addition to treatments for cancer and heart disease) found that while both research effort and spend have been increasing aggressively year over year, new insights are, conversely, sharply declining.1 So certainly there is an opportunity to improve efficiency here. Is AI the answer?
At first glance, it doesn’t seem so. We find that across life sciences fields of research, there has only been a moderate increase in AI-related publications since 1970 (especially in comparison to computer science and business management). Of all research fields surveyed, “pharmacology, toxicology and pharmaceutics” (research on the clinical behavior, pharmacokinetics, chemical properties and associated toxicity of drugs) saw the lowest rate of increase of AI publishing intensity. Here, the percentage of research utilizing AI only reached 2.2% after over 50+ years (see Figure 1).2
Which begs the question “why”: If 64% of businesses said in 2023 that they expect AI to increase their overall productivity — and science is a field that is experiencing a decrease in productivity despite an aggressive increase in resource allocation — why have we not seen an AI boom in science, especially in pharma?3
Figure 1: AI publishing intensity by research field (%)2.
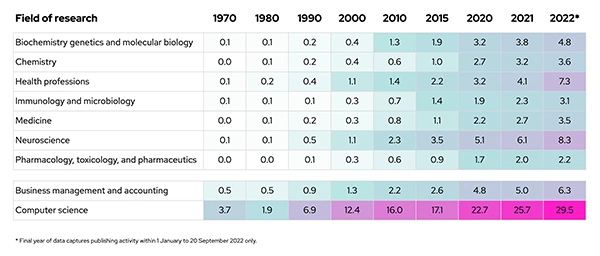
AI 101: What actually is AI?
AI is a very broad term, but there are a lot of things that AI is not: AI is not automation, it’s not a statistical model, and it’s not a pre-programmed software or a rule-based system. No matter what a vendor or supplier states, if what you’re using does not have the ability to learn or improve over time from new data, then it is not AI. So, then what actually is AI? When people say AI, they’re typically referring to artificial narrow intelligence (ANI). This includes subfields that are often referred to interchangeably — eg, “machine learning”, “deep learning” and “neural networks” — but in reality, there are nuances between these subfields. Generative AI (the form of AI popularized by ChatGPT and DALL-E), although powerful, is still considered ANI.
Memorizing classifications or some Venn diagram of AI subfields is not crucial to solving the mystery of a shortfall of AI adoption in science: The answer to our “why” question (“why have we not seen an AI boom in science, especially in pharma?”) lies in how an AI model achieves a prediction.
Black-box AI instils little confidence in scientists because it cannot answer "why" questions.
Most forms of AI are good at generating an accurate prediction, but they are not good at explaining “the why” behind their predictions. This is why AI is often referred to as a “black box”. Such predictions are generated when data is fed through thousands or even millions of interconnected layers of nodes that form a network. A single node may be connected to several nodes in the layers before and after it. It’s a small comfort that data is “fed forward”, meaning that data only moves through these interconnected layers of nodes in one direction. It’s still an immensely complicated and tangled system that is impossible to unravel.
Figure 2: An example of a very small neural network.
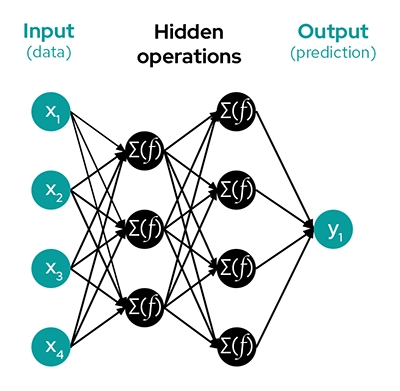
In order to squeeze out every bit of predictability possible from these millions of entangled and interlaced nodes, black-box models require you to feed them more and more data (also known as being “data hungry”). The data will first travel randomized routes through the network, and then the same routes over and over and over again as you “train” it. Every data point you feed it will go through this process, whether or not it’s actually important to the prediction. This is why at the end of the process, you’re left with only a prediction (because a very tangled ball of millions of routes does not provide you much value).
An example using black-box AI.
Let’s say you wanted to optimize lead candidates by modeling drug properties such as efficacy and toxicity. Black-box AI would be good at generating a single, accurate prediction (“active, no” or “toxic, yes”). But if that prediction wasn’t what you were looking for, you wouldn’t be able to determine the route travelled to arrive at that answer. You would simply have to try again and again with more data until you received your desired prediction (“active, yes” or “toxic, no”).
This is an example of just one problem in using black-box AI: A lack of explainability. For a scientist with a “why” question (“why is this compound active?” or “why is this compound toxic?”), a black-box model will never be able to explain why. To further complicate things, these models perform best when they’re trained to deliver a singular prediction. This introduces a second problem in using black-box AI: It’s difficult to combine models.
This means you might develop a model that is optimized for active compounds, but because you don’t understand the underlying mechanisms that lead to active and safe compounds, this model may as a consequence also be optimized for toxicity.
Scientists ask "why" questions. Can AI ever answer "why"?
A lack of explainability in black-box AI is why we don’t see significant AI adoption in science, especially in pharma.
Scientists ask “why” questions. Only knowing that a molecule is toxic is a one-dimensional finding; but knowing why a molecule is toxic to the liver — that is new knowledge. Understanding that a single mechanism might contribute to multiple outcomes (activity and toxicity) is a wealth of new knowledge.
If unravelling the mysteries behind chemical and biological interactions is our goal, then pharma needs AI that can offer explanations in addition to accurate predictions. Such AI is called “symbolic AI.”
Figure 3: Venn diagram of some symbolic sub-symbolic AI methodologies. There are, of course, many more varieties and subfields.
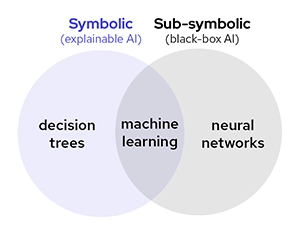
Why can symbolic AI answer "why" questions, while sub-symbolic AI cannot?
An example using symbolic AI.
Using the same scenario as before: Let’s say you wanted to optimize lead candidates by modeling drug properties such as efficacy and toxicity. Symbolic AI would be good at generating an explanation in addition to an accurate prediction, and it would produce a model that looks something like Figure 4.
Figure 4: An example symbolic AI algorithm.
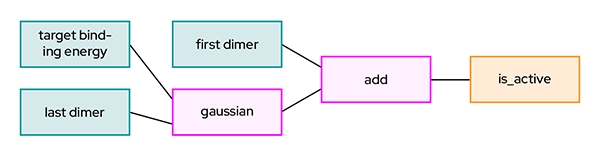
A scientist would read the model in this way: “There is a normal probability distribution (gaussian) relationship between target binding energy and last dimers. I can predict the activity of a molecule if I consider that in addition to the first dimer.”
Now this is new knowledge, and it’s an explainable, understandable phenomenon, not just a prediction. A scientist can confirm that hypothesis in published literature, or perhaps it can serve as a new hypothesis to be tested. It is the icing on the proverbial cake that symbolic AI requires much less data to come to this logical conclusion.
Symbolic AI is the path forward for science.
We must never forget that AI is a tool and like every tool, there are many varieties available — even within the same type of tool. Good application requires understanding your use case and your desired outcome and if your desired outcome is an explanation in addition to an outcome, then look to symbolic AI.
Pharma, and numerous other scientific fields, can access a wealth of new knowledge with AI. But like all good scientists, the AI must be able to answer “why” questions.
References:
1. Bloom N et al (2020) ‘Are Ideas Getting Harder to Find’? American Economic Review. 110(4): 1104-44.
2. Hajkowicz S et al (2022) ‘Artificial Intelligence for Science – Adoption Trends and Future Development Pathways’ Munich Personal RePEc Archive. 115464.
3. Visit: https://www.forbes.com/advisor/business/ai-statistics/.
4. Visit: https://en.wikipedia.org/wiki/Symbolic_artificial_intelligence.