Techtopia is available in Danish on Spotify, Apple Podcasts, PlayerFM, and IDA. The transcript has been translated into English and has minor edits to improve readability.
Om Techtopia:
Techtopia er en ugentlig podcast om mennesker og teknologi. Podcasten undersøger nysgerrigt emner om alt fra AI, blockchain, bio hacking, digitalisering, kvantecomputere, robotter og meget mere. Vært er Henrik Føhns, hidtil kendt fra DR Harddisken.
Henrik:
What’s really going on inside the machine when a neural network is working? How does a neural network, that is, an artificial intelligence, arrive at the result that comes out to us humans on the other side of the screen?
Yes, you can actually be in doubt about that sometimes. This is what is called black box AI. And the Danish company, the Danish startup Abzu, will do away with this. They want to do what they call ‘explainable AI’, a result that can be explained and understood, and for Abzu it is very much about issues in biotechnology and medicine development.
Abzu is an unusual artificial intelligence company, part Danish and partly Spanish as well. It is located in Barcelona, but mainly out on Orientkaj, out in Nordhavn, in Copenhagen. The wind is blowing quite strongly out there, but that’s also blown around the ideas they have. They are quite unusual. So I went out and met the company’s CEO and co-founder Casper Wilstrup. And first of all, we just had to have an explanation for why the company is called Abzu.
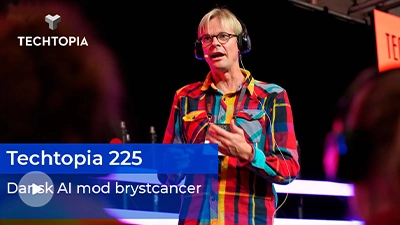
Casper:
I have a nerdy hobby, which is about studying Near Eastern culture and ancient culture. I have always been particularly interested in a particular grouping in Mesopotamia called the Sumerians.
The Sumerians were the ones who invented the written language. Not many people know that, for some reason people think it was the Egyptians. There isn’t much to suggest this, though. The Sumerians invented it first, and they made something called cuneiform.
Cuneiforms are those clay tablets that you probably remember seeing. I have always been incredibly interested in Sumerian culture, but also in cuneiform, and I have partly taught myself to read Sumerian clay tablets. One thing that you, of course, read a lot about when reading Sumerian original literature, is their religious worldview. And “Abzu” is the underground sea from which the world sprang. At least in early Sumerian literature. So in that way one can say that Abzu means the underground sea from which everything springs.
The company was not originally called “Abzu” in my head. I had made a prototype that I had called “Lib Abzu,” because it was the underlying library of our code. And when we were gathered somewhere in the Pyrenees, me and the original founding group, we thought, “What are we really going to call this company?” And had creative names like “Machine Cognition Lab,” and so on. And then there was one of my colleagues who said, “Let’s just call it Abzu.” At first I was a little “Abzu? Who can say “Abzu”? Nobody knows what that means.” But I ended up thinking that it had a certain beauty to use a name that comes from something I actually have as a very passionate hobby, and have had for many, many years. So: Abzu, the underground sea, from which everything springs.
Henrik:
At first glance, you may think it’s German.
Casper:
We had not even thought about that ourselves when we chose the company name. But we have been made aware since then that it also means “to and from” in German. But that is a coincidence.
My name is Casper Wilstrup. I am the CEO of this Danish-Spanish startup company which is called Abzu. At Abzu, we work with artificial intelligence. I have been very interested in artificial intelligence and the work of artificial intelligence for the last many, many years. Actually, I have a background in physics, but found an overlap between physics and artificial intelligence (at least on the technological level). I have spent the last 20 years of my life in various start-up companies.
But in 2018, I co-founded Abzu with some of the best high-performance computing and AI people whom I have come to know over the years.
Henrik:
You got into it a little bit by yourself because you say that you are dealing with artificial intelligence. Often when I go out and talk to startups, I usually ask first: What problem do you solve?
Casper:
So almost all artificial intelligence that exists today is designed to predict based on data and not with explanation as the focus. And that means that AI today is really, really good at being able to predict what we buy next time on Amazon, or which movie we feel like watching on Netflix next time. But when it comes to explaining phenomena, or when it comes to describing why certain types of things happen, most types of artificial intelligence actually fall short because they are simply designed with only results in mind. And what we had as our ambition when we founded Abzu was to start over and say, “Let’s make an artificial intelligence which is built from the ground up to provide explanations instead of just predictions”.
Truth is, if you have a really good explanation for a phenomenon, then you can also predict what is going to happen. But just because you can predict what’s going to happen does not mean you have an explanation for the phenomenon.
In a recent interview I did, I talked about how I think one can compare many of the artificial intelligence techniques that exist today with oracles: We train those sitting on data and then we can ask them questions and they can give us an answer. But what they can answer is only “what will happen”, not “why things are happening”.
It fundamentally leaves a gap, especially in relation to research, so we actually abandon research in the traditional sense where we want to understand the world we live in, in favor of a confidence that the computer can predict what’s going to happen. We can go a long way with that, but along the way with that approach we will basically find that scientific progress will come to a standstill. It worried me and our founders, and I had a dream that we could build a technology that could simply address that problem head on. And we have succeeded in that.
Today we have invented an AI that focuses on providing explanations: “Why do things happen?” “Why is a new medicine toxic?” Not whether it is toxic, but, “Why is it toxic?”
Why does someone die of cancer, while others survive? Not whether they are going to die from it, but why they are dying from it. Because in the answer to the “why” question, there is also the first step to a solution.
So the idea for what we do, it was actually an idea I got all the way back in the 90’s. I was a student at the Niels Bohr Institute, and I worked on building computer clusters, i.e. large groups of computers that were connected in such a way that you could run large simulations. This is something that is especially used for quantum field simulations. And there I got the idea that some of these methods could also be used to look for mathematical explanations in data, rather than just looking for models that can predict, which is what the neural network is basically about. I didn’t do much about it at that time, maybe the computer resources were not really ready for it back in the mid 90’s. But I kind of had the idea all along; that there was a fundamentally different approach to artificial intelligence that we could address by thinking it in from the beginning in the design of our systems.
So we built our technology, we call it the QLattice®, referring to the fact that it has its origins in some methods that come from quantum field theory. But the technology is designed from the ground up to provide the simplest possible explanations. One way I sometimes explain it is that if I show some pictures of a bus, then the technology can see that it is a bus and that is what the neural network is super good for.
But if I ask you how many red cars there are in Spain, then a cognitive process starts. You might be thinking, “How many people are there in Spain, and how many of them have cars, and what percentage of the cars are red?” And then you kind of get through a thought process that can come up with a suggestion. And what our technology is designed for is to make this thought process. So one can say that the way to solve this question is simply by starting to ask oneself, “How many people are there in Spain and how many of them have cars?,” and so on. So the system we have built is looking for the explanations of how to arrive at answers rather than the answers themselves. And neural networks do not. They are simply designed for giving answers, so therefore we have some unique properties with the technology we have built that makes it particularly relevant in some contexts, and especially when one is either interested in research, i.e. theories, or when you need it for some other reason to be able to explain why the computer says what it says.
Henrik:
I don’t assume that you use the technology to find red cars in Spain, even though you have an office in Barcelona. So what do you use the technology for? What can you do?
Casper:
Yes, so we are a small, relatively new company. We will soon have four years behind us. We are around 30 people. We have invented a technology that can be used in virtually any business area where explanations are needed. But we have chosen to focus a bit – which you have to do when you are a small company – on health research and medical research.
So both our academic work and our clients are involved with understanding diseases and using the knowledge about diseases to be able to select and design new forms of treatment that can cure those diseases. So we both go in and work on, for example, understanding some of the mechanisms that lead to cancer. It is a very active topic in our company and among our collaborators, but we also think about the fact that once we have understood the mechanisms behind cancer then we can then use the new understanding to design some types of medicine or other forms of treatment which can better deal with the cancer. So we have these two sides in our work: One is health research, medical disease understanding basically, and the other is treatment. How can we design some typical drugs that can handle this situation better, so that patients get a better outcome?
Henrik:
And the reason you can design medicine is because you know the causes through the second part of your work?
Casper:
Yes, so an example: We have worked a lot with breast cancer, where by analyzing some data from about 700 women with breast cancer, we arrived at a relatively simple explanation that involved two specific genes which have a very large significance for whether women survive or die of their cancer. And if they have certain levels of these two genes, then their risk of dying is much greater than if they didn’t. And once one has understood such a connection – two specific genes that are set aside for some proteins in the body – then you also have a way to go in and regulate it.
All of a sudden you know that if you can reduce this gene down, one of the two genes in question here is something called APOB, and you can reduce the APOB level down, then the probability of the woman surviving her cancer simply gets far greater. So now all of a sudden we have what’s called a “druggable target”. We know that something can be done here, so the next mission is of course to find out how we can make a type of medicine that actually reduces the level of APOB. You can then go into all possible levels: You can go in at gene therapy level and you can go in with RNA medicine and you can go in with molecular medicine or maybe some peptides, which are also called proteins. So there are many different ways and then one can start researching it. It is, of course, a long process.
I am not promising that just because we come up with good new explanations today, which are actually quite significant in understanding different types of cancer and also other diseases, then of course it takes some years before it has been converted to types of medicine, which can then actually help in these situations. But as I said, we work a lot in both fields because there are explanatory issues in both situations.
First you will understand the disease and once you have understood it, then you can develop some types of medicine that might help. Then you try them out, typically first in some simulations or in some single cells and later maybe on living organisms. And then it often turns out that they do not work the way you think they do. They are maybe toxic or they just do not have the effect on the gene you would like to down-regulate.
And then you will say, with typical machine learning, which is what the pharmaceutical industry today will use, you can go in and try to model it with neural networks. For example, we can now predict which drugs are toxic, but with our technology, we can ask the question differently and say, “Why are these drugs are toxic?” And when you have the answer to a “why” question, well then it is also much easier to prevent it from happening than if you just have a “brute-force oracular predictor” who can tell you what is going to happen sometime.
Henrik:
You said 700 women with breast cancer. So 700 is a very small data set compared to the fact that you always hear that artificial intelligence can process huge data sets and oversee things that we humans can not see. So what’s the benefit of using such small datasets? And why does it work when you usually have to use very large data sets? I don’t quite understand.
Casper:
No, that’s a really good question. So neural networks are especially very, very data hungry. In fact, they only work if you have tens of thousands or hundreds of thousands of observations, so an issue like this would be really difficult to address with neural networks.
The reason our method works is that we are looking for simple explanations. Simple explanations can be found in smaller datasets, rather than very complicated explanations.
So when you have 700 women, then you can not find an explanation with a neural network. If you have 700 women and have segmented their full genome, then 3 billion is the number of base pairs. If you want to say, “What is the variation in these women?”, that is, the probability that they will have this accident, the bad outcome. Well then it can not be done. Then you should collect data on millions of women who have died of breast cancer. Fortunately, this is difficult. So our type of technology is simply designed to find simpler explanations, and that is the principle called Occam’s Razor. They are more likely to be correct even if they are based on fewer observations. This is how science has operated for millennia, in fact: simplest possible explanations based on data. And that makes us have an advantage here.
At the same time, our technology delivers explanations, not predictions. So when the explanation comes out and says, “Well, it seems that these two genes, in an inappropriate combination, cause faster growth of cancer and therefore greater risk of death,” then the researcher who sits and uses our system can say, “This seems familiar. Let me just look up the APOB gene in this knowledge database and see what else we know about APOB,” and discover that there is already some research here from researchers who have collaborated with them here and who have found that it also plays a role in liver cancer.
Then they take some of that knowledge and then all of a sudden we activate the researcher’s head instead of just providing the answer on a silver platter. And it also allows the researcher to eliminate the bad explanations, if there are bad explanations in the mix, of what comes out of our technology. Then you can just think about whether this relationship makes sense, and it makes a huge difference for all researchers.
Sometimes we say that black box AI is about replacing human thinking, and our AI is about strengthening human thinking. So we do not see ourselves as something that goes in and does the work for people. We are extra brain capacity, which makes it easier for people to get the ideas that they can then further develop.
So as a researcher, the experience is that you sit with a data set and you are presented with some hypotheses, as it is called in the world of science. As a researcher, you can relate to these hypotheses yourself and say, “Which of these hypotheses do I want to go further with? Which one should I use in a new experiment? Maybe I should set up an experiment where I see how APOB works in reality in single cell experiments?” So we are a tool in the traditional research method, rather than a substitute for the traditional research method. In my opinion, it is a wrong replacement. Black box AI is leading nowhere, but that’s a bit of a different talk.
Henrik:
Maybe a bit of a silly question, but can you compare yourself to Gyro Gearloose’s Thinking Cap?
Casper:
Yes, actually! Yes, it is actually a very good comparison. Gyro Gearloose’s Thinking Cap makes him think better. It does not replace him, so I like that context.
Henrik:
You’ve mentioned the work with the 700 breast cancer patients. What else have you done?
Casper:
We have worked with so many different diseases: Alzheimer’s, preeclampsia, liver cancer, etc.
I myself have worked a lot with preeclampsia, which is a disorder that affects pregnant women. Fortunately, it is not as serious as breast cancer. But still relatively serious and cost many fetal lives every year. Well, maybe not many, but too many. So we have worked to understand some of the mechanisms that cause some women to develop this condition. Preeclampsia is characterized by very high blood pressure and sometimes this results with one having to terminate the pregnancy.
But basically, we outsource the tool to some researchers, and sometimes we are personally involved. I mention preeclampsia here, because I have been personally involved in the project. Other times, there are some researchers who work with the technology on their own. So there are hundreds of diseases that have been studied using our technology.
I think as an overarching theme, we have a relatively large interest in cancer. This is an area where our technology is very suitable, because there are difficult questions where it’s often a bit like looking for a needle in a haystack to understand the many, many, many different types of cancer. But by having a technology like ours, you become really good at looking for this needle in a haystack. So it is an issue where our technology is particularly suitable, so we see a lot of it.
Henrik:
But is it particularly suitable for smaller diseases? That is, where there are not so many cases or diseases that are very rare?
Casper:
It’s definitely where the difference is greatest, because if you have relatively rare diseases, then you also have relatively small data sets and the common machine learning technologies that are out there fall short with small data sets.
Now you say 700 is a small dataset, yes, but the preeclampsia dataset is thankfully even smaller. Or some of the other diseases we have worked with, we are down to a hundred or 80 patients sometimes, and that is the explanation space you work in. It consists of the whole human genome, if you try to understand why some humans develop a particular disease and we think maybe there is some genetic explanation. But you have so many genes. We as humans have so many genes. How can you do that? It’s a very large space to search through, and neural networks, for example, just can’t do that.
So you could say that we have a unique advantage in that we are the only way to approach problems when your dataset is so small. But even when the datasets are large and even with the very large diseases, there is no doubt that our technology still has the central advantage that it provides explanations in addition to a prediction. I always think it’s relevant, no matter how big the dataset is.
Henrik:
It may somehow sound like you’ve invented this thing, and then it’s just enormously easy to drive through these datasets, but I guess it isn’t? What is the cost of this? Is it an expensive technology you use?
Casper:
Well, the actual technology, it’s relatively computer-intensive, so we’re like other AI machine technologies in that it requires some pretty powerful computers. That is also why it has only become possible now.
Henrik:
But what does it require?
Casper:
Most of our analyses run on a cluster, which consists of 15-20 ordinary computers, physically located in Germany. So it really isn’t bigger than that. But then it might take half an hour to an hour, sometimes half a day, to analyze a data set. If we had some bigger computers, then it could go a little faster. So in that context, we do not differ from other machine learning technologies, but it is clear that it is something that one must take into account.
But now that you asked where the barriers are. Why have we not just answered the question on all these diseases? It’s not about algorithms or computer power. It’s about data. So in the end, if we want to understand diseases faster and become better at treating them, then it’s also about getting that data together and making it available to algorithms so that we can actually start to ask the questions to the data.
Today, that is the biggest obstacle in moving forward. There are basically two challenges. There are the regulatory challenges, which are about privacy and there is a lot of respect for that, but you can solve that by saying that we move the algorithm to the data, instead of the data to the algorithm. Our technology is basically designed for this same reason.
But in addition, it is also about data collection and getting correct data. After all, it is not super easy to collect data on patients with a relatively rare cancer disease. It takes many years. You need to monitor them, gather them, and get these gene tests taken. And if it’s a genetic route you want to look for, then you need to sequence their genome and so on. So it is both time consuming and expensive and, incidentally, also relatively often fraught with many errors, the process that leads to data. This is the biggest challenge to really push something on health research.
Henrik:
So basically it’s the data collection and ensuring uniform data and so on, and ensuring the data you need exists?
Casper:
When it comes to biological systems, yes, it is. But it is also the part that is about understanding the diseases.
To treat the diseases, that’s another conversation, because you can generate data in the laboratory. Because there you can generate some new molecules, and then say, “And how do they work on a single cell?” So you don’t have to go out and wait for people to get sick. There you can simulate it in different ways, for example within computer simulations or in individual cells. So a lot is going to happen in research both in disease understanding, but also treatment of diseases in these years.
And it’s exciting for us as a company to be in the heart of this, and just see how fast it really goes.
Henrik:
When you say that you move the algorithm to the data, you mean you analyze the data where the data is so they do not have to hand their data out. So people’s identities are not revealed either.
Casper:
Yes, well, there is a recurring theme in the way we think at Abzu, and that is regarding transparency and ethics, but also privacy. We try to include such relevant considerations in the work we do.
So from the very beginning we have designed our technology and our algorithm to be able to work in a way where you have to respect people’s data privacy. So you could say that the ethical aspect of the way we think is both that we think it’s more ethical to explain things than just provide a black box prediction, but it is also about being able to integrate the understanding of data by going to the data and analyzing there, so you don’t have to make these very large collections of data.
For example, I think it is a relatively big threat or risk to the future of us all that we gather huge genetic databases together about all Danes in some central data warehouses. It’s only a matter of time before it gets out, and then a lot of things can be done with our genetic data, which I am personally quite worried about. So part of the solution is not to gather data together in huge data centers. But instead moving the analysis of data out where it is, as we are then much less vulnerable to data security issues as a society.
Henrik:
So you mean our data, our health data, should be stored in different places?
Casper:
Yes, I think so. Basically, distributed data storage is much more robust. A total breach of some of the data centers we have in Denmark and then it’s game over, right?
So if we have gathered the genomes of all Danes in a central database, and if it is compromised, then it is really compromised. If it is local storage out in the individual places, if we can find good methods for this, then we can analyze it without putting it all together in one place, then the system is much more robust.
I basically believe that we as a society should think much more about transitioning to distributed data storage for damage minimization reasons.
Henrik:
If it does happen and someone would compromise the data, what is the risk? What can it really be used for? Because the difference between you and me is not really that big. Maybe there are some minor differences that make me have an illness that you do not have or vice versa. But what is the risk really?
Casper:
I probably have two answers to that: one is that I could come up with some examples of a risk around the personal data about us. If you can predict things based on the individual’s genome, then it can be misused in many contexts. After all, it is a societal decision whether we want to abuse it. And it is also a societal task to prevent abuse, because in the end, genome understanding is out there, and we as a society have to ensure that it is not abused for, for example, profiling in relation to insurance or financial services or prioritization of health care, based on who may have the most to gain from this and other things we ethically do not like. It is our decision as a society to decide what is ethical and what is unethical. Some of it can be solved by regulating abuse. Basically we say, “It’s not because we’re going to make it impossible to do. We’re just going to make it illegal to do that.”
We use it a lot, for example in the insurance industry: You can include these parameters when deciding what people’s insurance premiums should be, and you must not include these other parameters.
But it could also be that this data ends up in the hands of people who do not feel subject to regulatory requirements of this kind, and who can use this in all possible ways in relation to having a go at us with opinion-creating material on the Internet or any other method. Or sometime in the future, well, I do not want to be a dystopian and say that we will have a surveillance society, but if we ended up having a society where the authorities had more power than they should have, then it might also be very nice that they at least did not know our genome.
But basically, the risk of health data profiling is hypothetical. There is no doubt that it can be abused. But what is really important is whether we are going to allow that abuse. But by not having it spread out in the big world as a whole, there is at least some kind of safeguarding against this abuse.
Henrik:
Now we are moving on to a more ‘monitoring’ discussion which is really about not knowing what kind of government you have tomorrow, and if you have given some permits now, then you have to keep in mind that they also apply in the future.
Casper:
For me, it’s crucial that our technology puts science back into the driver’s seat. I think for the last 20 to 30 years we have seen a tendency to give up on theory. Now we just gather data together and build these supercomputing AI methods, and then we just connect the two things together – AI and Big Data – and then we can answer all sorts of questions.
And if Sir Isaac Newton or Galileo had done that, we would not have made it anywhere. It may well be that Galileo had used his supercomputer to calculate how long it took before the feather or stone hit the ground, but if he had not set out the understandings he made in theoretical form, then we would not have progressed from there.
I think we forget that, and in my opinion, it’s of the utmost priority to bring theory back to the center of science. But on the more down-to-earth level, explainable AI also has another important ability. It enables the users of the technology to explain to people why they are making the decisions they are making. “Computer says no, computer says yes” is in many contexts not the right answer. Why can this user get this credit rating and this other user get this credit rating?
So it’s really nice, both for the customer themselves, but also for the credit institution, to say, “Because this, this, and this”, because otherwise a suspicion quickly arises that there are some unpleasant biases involved in some of these decisions. Sometimes there actually is, and even without anyone knowing it. So by bringing transparency forward, as a method like ours does, it becomes obvious to everyone what the model makes its decision based on, because it is there, revealed to your eyes: “This is the explanatory model that we have used to make the decision for your credit rating.” This applies to a broad ratio of subjects.
If I had to know that I was not allowed to get a certain form of chemotherapy while someone who had the same cancer as me was allowed to get it, then I would really like to know why. And it is certainly not nice to hear that there is some neural network that has examined my genome and said that it is not very smart to give it to me. Then I would actually rather know that it is because this kind of treatment does not work on people who have the wrong level of this gene here, and I have that unfortunately. OK, then I may die. But at least I know why it was that this medicine would not work on me.
So in many contexts, it is a prerequisite for an actual ethical decision-making process that one can explain one’s decisions. And I think there is too much reliance on traditional AI methods, and neural networks can risk taking that understanding from us. That being said, there are plenty of uses for neural networks and black box AI that are completely responsible and sensible and super value-creating, so it’s not because one excludes the other.
I like a wording which economist and psychologist Daniel Kahneman, which many may have heard of, has described as System 1 and System 2. I think traditional AI and neural networks are similar to System 1 in Daniel Kahneman’s model, while the kind of AI we do is similar to that of System 2. And there’s room for both. There is room for these fast data-based decisions that neural networks can deliver, and there is room for the more rational, considered, and reason-driven forms of decisions that System 2 in Daniel Kahneman’s model can deliver. So it’s not “either / or”, but more “as well as.”
Henrik:
That was Casper Wilstrup, CEO and co-founder of Abzu, an artificial intelligence company that makes explainable AI. This interview marks the beginning of a series that we intend to make here at Techtopia, which deals with artificial intelligence in the health sector. We will revisit this subject over the next few months.