Krishnaraj Chadaga, Srikanth Prabhu, Niranjana Sampathila, Rajagopala Chadaga, Muralidhar Bairy, and Swathi K. S.
Abstract.
Child sexual abuse (CSA) is a type of abuse in which an individual exploits a kid/adolescent sexually. CSA can happen in several places, such as schools, households, hostels, and other public spaces. However, a large number of people, including parents, do not have an awareness of this sensitive issue. Artificial intelligence (AI) and machine learning (ML) are being used in various disciplines in the modern era. Hence, supervised machine learning models have been used to predict child sexual abuse awareness in this study. The dataset contains answers provided by 3002 people regarding CSA. A questionnaire dataset obtained though crowdsourcing has been used to predict a person’s knowledge level regarding sexual abuse in children. Heterogenous ML and deep learning models have been used to make accurate predictions. To demystify the decisions made by the models, explainable artificial intelligence (XAI) techniques have also been utilized. XAI helps in making the models more interpretable, decipherable, and transparent. Four XAI techniques: Shapley additive values (SHAP), Eli5, the QLattice, and local interpretable model-agnostic explanations (LIME), have been utilized to demystify the models. Among all the classifiers, the final stacked model obtained the best results with an accuracy of 94% for the test dataset. The excellent results demonstrated by the classifiers point to the use of artificial intelligence in preventing child sexual abuse by making people aware of it. The models can be used real time in facilities such as schools, hospitals, and other places to increase awareness among people regarding sexual abuse in children.
Explainable Artificial Intelligence (XAI) to Interpret Results.
…
The QLattice is an interpretable framework for ML models (Wenninger et al., 2022). The QLattice explores many models before settling on the one that best fits the problem. The programmers must first set up a few parameters, including input properties, variables, and other labels. In the QLattice, the variables are named registers. Models are constructed after the registers are specified. The newly generated model is known as “QGraph” which consists of nodes and edges. Every node has an activation function and a weight assigned to it. The QLattice is implanted using the “Feyn” library in Python (Riyantoko & Diyasa, 2021).
Figure 14 depicts a QGraph generated by the QLattice model. Green nodes represent inputs and output. The nodes in white with pink borders depict an interaction. Interactions process input values, construct functions with various transformations, and predict the final output. According to the QLattice, the most important attributes are “Do you know what child grooming is?,” “Children are safe among family members such as grandparents, uncles, aunts, cousins,” and “Do you know what signs to look for to identify if your child has been abused?.” The transfer function used by the QLattice model is described below.
The most important features according to XAI techniques were “Do you know what child grooming is?” and “Do you know what signs to look for to identify if your child has been abused?.” The above two questions were highly useful for the classifiers to distinguish between the two classes.
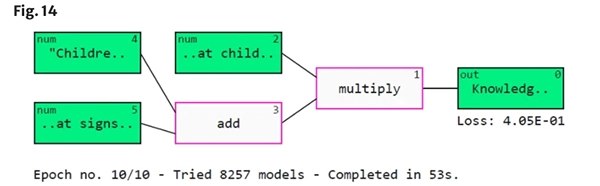